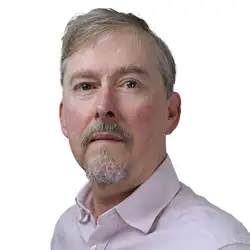
Written by Dr Peter Debenham
Senior Consultant
Evolving silicon choices in the AI age
Only a few years ago choosing silicon for a processing job seemed simple.
Either use a CPU or GPU (if your task had a lot of parallel sections) or possibly create a totally bespoke FPGA or ASIC (much more expensive in engineering effort but often worthwhile in many high value jobs).
Now it is much more difficult. The old options still exist but new options exist such as TPUs (Tensor Processing Units) and their cousins NPUs (Neural Processing Units). What changed?
The change of course is Artificial Intelligence (AI). In the past few years AI has changed from a subject only talked about dryly in academic journals or otherwise displayed in Science Fiction where, usually, the AI is out to kill people in various ways (e.g. Ava in Ex-Machina 2014, Schwarzenegger’s Terminator 1984, or HAL from Kubrick’s 2001: A Space Odyssey 1968 – all three great films by the way).
Artificial Intelligence in the real world is taking a complicated set of inputs (pixels of a picture maybe), applying a series of weights or biases to those pixels across a number of layers to get a simple output (picture contains a person and a cat). Performing any single set of calculations is not usually too slow for modern computers but away from those dry academic journals most people want to perform a lot of sets of calculations and perform them fast. People want some kind of real-time response to a changing situation, say frames from a video camera but to achieve this they generally would prefer something which is physically small, efficient, and not doubling up as a fan-heater – silicon and electricity cost money after all even when you are plugged into the mains.
If the situation is computationally intensive enough when running a trained AI it is even worse when attempting to train an Artificial Intelligence model. This is an iterative process. A set of carefully chosen training data is fed through a putative AI model and the accuracy of the resulting output is measured. Based on how well the model performs changes are made to the model and the process is repeated. Training a large AI model requires using a large set of carefully chosen training data and many cycles of the loop; process data, check accuracy, refine model. Essentially the training process must run the AI model an enormous number of times. Whilst silicon cost and power consumption remain a concern the big driver here is usually the elapsed time necessary to train the model which can easily be tens of hours or even tens of days. Reducing this is, relatively speaking, worth a lot of electricity and silicon cost especially where the silicon can be rented from Amazon, Google or similar.
Given the requirement to run AI models in a fast and efficient fashion what type of processing silicon should be used? For a fixed model it is possible that a bespoke FPGA is fastest and least power hungry. But the time and cost of designing and implementing a bespoke FPGA remains considerable and is unaffordable in most circumstances. Consider that AI development is moving fast enough that a good model today will be a bad model tomorrow and a bespoke FPGA even less likely to be a good solution.
The Central Processing Unit/Graphics Processing Unit (CPU/GPU) equation remains like before. A single CPU core can perform a general complicated set of mathematical operations very fast. Each core in a multi-cored CPU can perform these calculations almost totally independently of the other cores. A high-end processor such as the AMD Threadripper Pro 5995WX has 64 cores and particularly when training an AI, it is possible to devise algorithms which use each efficiently. Even a more mainstream, lower cost and power consumptions, Intel i7 may have 12 or more cores.
Conventional GPUs operate differently to CPUs. Rather than a lower number of very powerful processing units, cores, these contain vastly more numerous but less individually powerful cores. The Nvidia H100 GPU has 16896 cores. Even a “humble” GeForce home PC graphics card can have over 9000 cores. But there is a catch. As well as each core being less powerful than a CPU core, GPU cores cannot each operate individually. They are grouped together (often in groups of 32 or 64 cores) and each such group must operate in lockstep and do so without using the storage memory required by other groups. Where software can be written to make efficient use of this the system can run much faster than on a CPU due to the large number of cores. This has been done for many software packages which train common AI model architectures.
More recently Google created a new type of processor specifically designed around the needs of a particular type of machine learning AI, convolutional neural networks (CNN). This is the Tensor Processing Unit (TPU) which Google announced in 2016, though they had by then been using them in-house. TPU workloads are available as part of Google’s cloud architecture (cloud TPU) and use Google’s own TensorFlow software. The TPU is a bespoke ASIC designed for high throughput of low precision calculations, specifically matrix processing. TPUs were originally designed for running already trained CNN models where they are more power-efficient per-operation than the more general GPU or CPU but can (since version 2) be used additionally for training such models. Waymo, uses TPUs to train its self-driving software.
At a similar time Nvidia added Tensor Cores to its data centre GPUs (2017) and its consumer GPUs (2018) again targeting matrix multiplication and accumulation operations. This adds efficient AI acceleration alongside the other advantages GPUs have over CPUs. The data centre H100 GPU adds 528 Tensor cores to the 16896 Cuda cores. In 2022 Tensor cores started to appear in lower-power devices such as the Jetson Orin Nano.
In general GPUs are preferred to CPUs for running large AI model training workloads. Often the choice over which to use is not what is theoretically “best” but which hardware you have available either physically in a machine you control or are able to rent in the cloud. For much of the past few years those wishing to purchase high end GPUs have suffered from significant, many months long, backlogs as demand overwhelmed the supply chains. OpenAI used GPUs to train its large-scale AI models such as GPT-3.
Neural Processing Units (NPU) are like Google’s TPUs. They again offer hardware specifically designed to accelerate aspects of neural networks and AI. NPUs may be standalone data centre cards or integrated alongside CPUs both in PCs (Intel Core Ultra Series, AMD Ryzen 8040) and mobile (Qualcomm, Huawei) or even lower-power edge processors.
Of particular interest to Plextek is adding intelligence “at the edge” for Internet of Things (IoT) devices where per-unit cost, computing power, battery capacities and communication bandwidth are tightly limited. The option of transmitting everything to a more powerful server which can do the number crunching is not possible. This means the ability to run an AI model locally efficiently and rapidly on an IoT device is necessary for it to respond to its environment only as and when needed.
IoT devices in addition to its sensors typically need an embedded microcontroller. For moderately complicated devices this is often some version of an Arm Cortex. These are capable of running AI loads but are often too slow or too power hungry to be practical. Then some kind of AI accelerator is required to both speed up the time to run the model and reduce the energy required from the limited power budget.
Examples of currently available “at the edge” accelerators include Google’s Edge TPU (a smaller version of their datacentre TPUs), Arm’s own Helium technology (Armv8.1-M Cortex-M chips such as the Cortex-M85) and NXP’s eIQ Neutron NPU (Arm Cortex-M33 based MCX-N series). At the higher power end of IoT there are devices such as Nvidia’s Jetson Nano boards incorporating an Arm A57 and a 128-core Nvidia GPU (an older design lacking AI specific Tensor Cores) or the Orin Nano containing a more recent Tensor core supporting GPU.
How much faster are the accelerators compared to just using the conventional Arm core? Numbers can be hard to find but NXP and Google publish some public data. Google shows the inference time difference between an Arm A-53 core and its development board (Arm A-53 and Edge TPU) when running various AI models trained using the ImageNet dataset. The Edge TPU is typically 30+ times faster than the Arm core by itself. This comes with the limitation that the Edge TPU is only designed to process a limited range of AI model types.
NXP does not give precise numbers for inference time but shows “ML Operator Acceleration” from the NPU compared to just using the Arm-M33 core for three different typical AI operations. As with the Edge TPU acceleration is found to be around 30+ times.
Nvidia make the point that their GPU solution can process models which the Edge TPU cannot by showing frames-per-second the Nano can manage when running Classification and Object detection models with frequent DNRs (did not report) for the Coral Edge TPU development board. Where both platforms could run the model, their speeds were similar.
Which type of AI processing platform is the best? As the Nvidia blog shows this depends on precisely what type of AI you are trying to run. Some types of processing platform can only handle a restricted set of AI model types. Others provide acceleration to a wide variety of AI processing loads but are tightly coupled to manufacturer’s CPU cores. As the use of AI continues to increase the only certainty is that the CPU/GPU/TPU/NPU acronym list is going to continue to grow.
Technology Platforms
Plextek's 'white-label' technology platforms allow you to accelerate product development, streamline efficiencies, and access our extensive R&D expertise to suit your project needs.
-
01 Configurable mmWave Radar Module
Plextek’s PLX-T60 platform enables rapid development and deployment of custom mmWave radar solutions at scale and pace
-
02 Configurable IoT Framework
Plextek’s IoT framework enables rapid development and deployment of custom IoT solutions, particularly those requiring extended operation on battery power
-
03 Ubiquitous Radar
Plextek's Ubiquitous Radar will detect returns from many directions simultaneously and accurately, differentiating between drones and birds, and even determining the size and type of drone
Downloads
View All Downloads- PLX-T60 Configurable mmWave Radar Module
- PLX-U16 Ubiquitous Radar
- Configurable IOT Framework
- Cost Effective mmWave Radar Devices
- Connected Autonomous Mobility
- Drone Sensor Solutions for UAV & Counter-UAV Awareness
- mmWave Sense & Avoid Radar for UAVs
- Exceptional technology for marine operations
- Infrastructure Monitoring