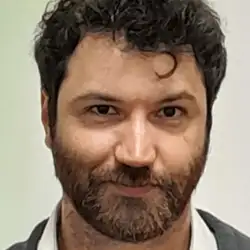
Written by Jason Pompeus
Senior Data Scientist
SSL: The Revolution Will Not Be Supervised
Leveraging expertise across the RF sensing, communications, and low SWaP (size, weight and power) electronics domain spaces, Plextek delivers custom R&D and product development across a range of industries including consumer, healthcare, and defence.
To ensure we remain positioned to best support our customers’ needs, Plextek prides itself on continuous innovation, and the conscious application of new and emerging technologies.
This includes the rapidly evolving field of machine learning (ML), where Plextek has demonstrated interdisciplinary expertise in finding novel solutions to new and existing problems. Using image generation architectures to rapidly model radio frequency (RF) propagation, exploring the use of transformer self-attention for interpretable data fusion, and classifying and parameterising RF modulation schemes for electronic countermeasure tasks are some examples of such technologies that Plextek has applied to real-world problems.
Here we look at a new training paradigm for machine learning architectures – called Self-Supervised Learning – which has the potential to improve the accessibility of ML approaches to niche and under-represented domains, while simultaneously reducing the time and effort spent on dataset curation. We explore the motivation behind this technique, how it works, and how Plextek can use this technology to continue innovating in an ever-evolving landscape.
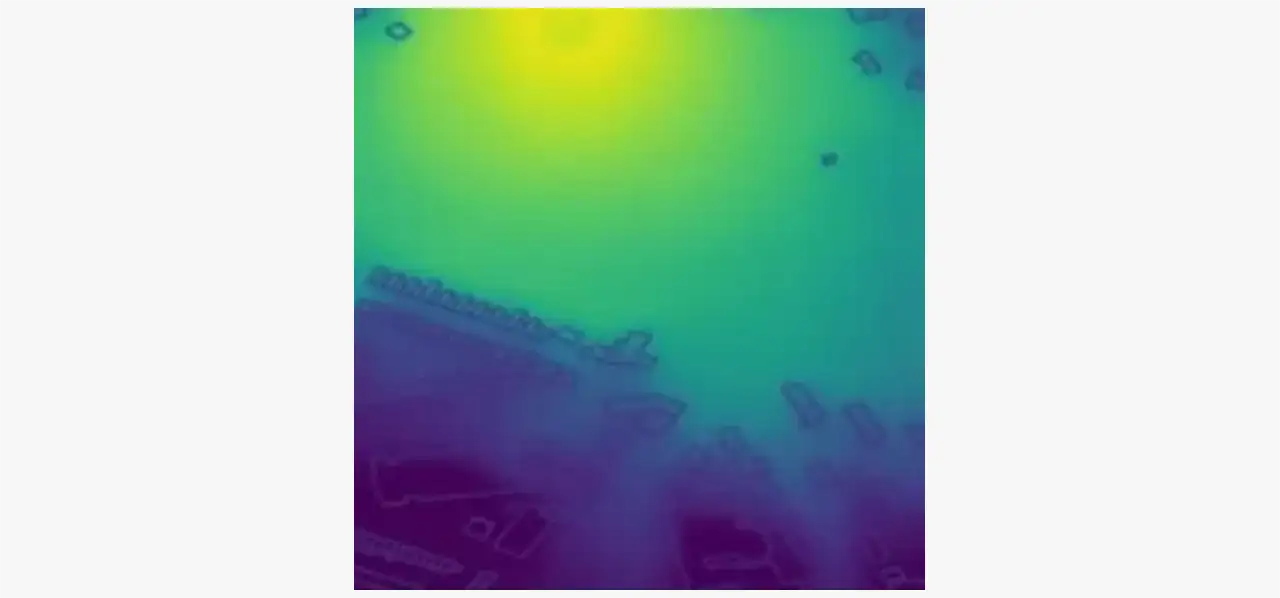
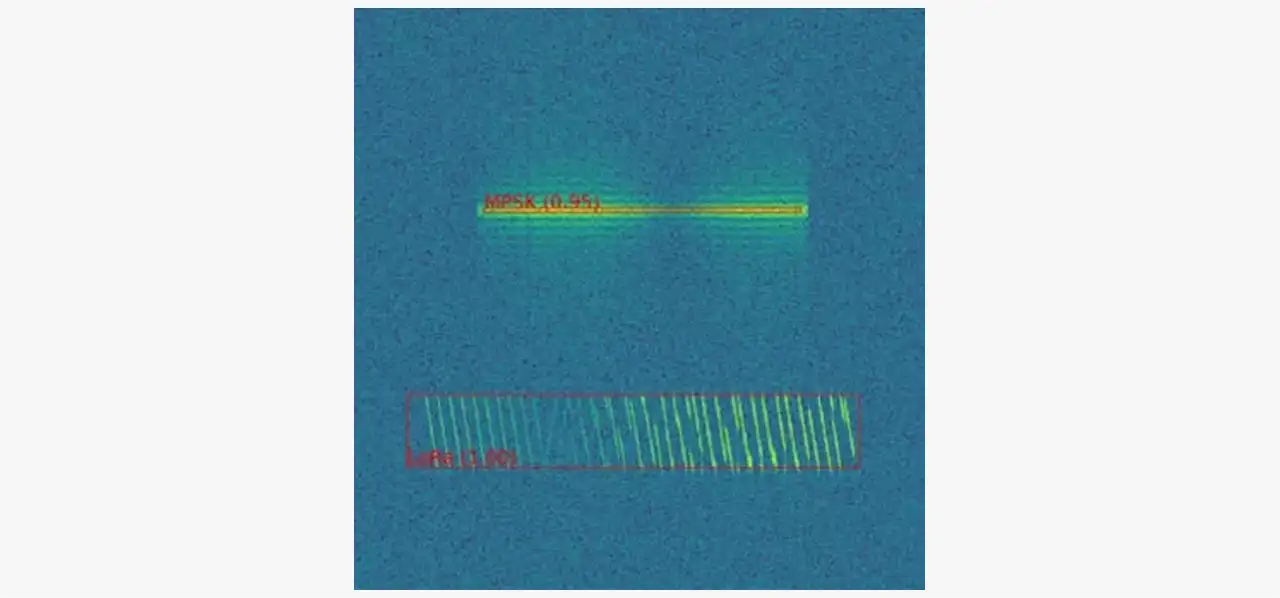
It’s a familiar paradigm of machine learning: if you want your model to perform – and to perform consistently – you need data, lots of it, and diverse enough to capture all the key features and corner cases your model will need to recognise once deployed. This volume of data needs also to be labelled. That is, the training framework needs to know a priori what each sample represents, to mark the model’s predictions as right or wrong, and to update its parameters accordingly. As models grow in complexity, so do they require vast and varied datasets, and so too do unlucky teams of analysts need to perform the laborious job of labelling it.
This method of training ML models on labelled datasets is known as supervised learning, and for expansive, increasingly multi-modal applications such as natural language processing, speech recognition, or generic object detection, the process of labelling your dataset can be a costly, tedious and time-consuming enterprise in its own right. But what if there were a way for your model to learn important features from the data without supervision, i.e. without labels? After all, human intelligence learns in large part from unsupervised observation of the world it inhabits – very young children, for example, learn their first words by listening to and imitating conversations around them, not from extensive and repeated lessons in grammar.
This is the core idea behind self-supervised learning (or SSL). SSL trains the model to learn useful features from an unlabeled dataset by maximising the similarities of internal representations of like data samples. Typically, this involves two neural networks operating in parallel – a student network and a teacher network – with matching architectures and shared weights.
By sharing weights, the networks are essentially twinned – given the same input sample, both the student and teacher would encode identical representations. However, during training, each network is presented with a different “view” of the same input sample, initially resulting in two unrelated representational encodings. A projection head at the output of each network maps the encodings back into a space where a contrastive loss function can be applied, and this loss function updates the weights of the student network to maximise the agreement between its output and that of the teacher. The new weights are then copied across to the teacher network, and after multiple iterations, the student learns a consistent encoding for the sample regardless of the “view” it has of it.
(Modern implementations perform a slightly more sophisticated approach, but the same general principle is followed).
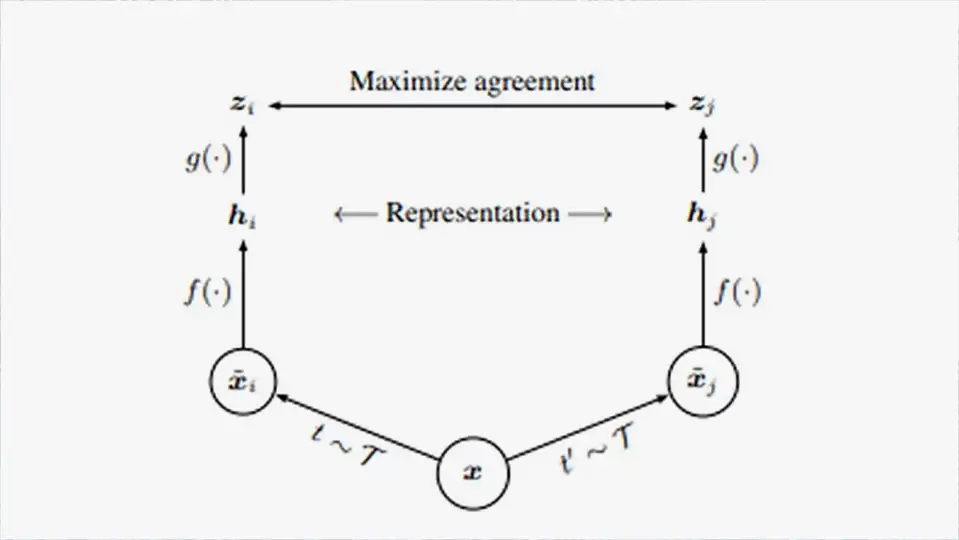
These “views” are generated by applying carefully chosen transforms to the data samples, that preserve the features of interest but change the context in which they appear. For example, for a large language model (LLM), the teacher may be presented with a sample sentence, and the student presented with the same sentence with one or more words removed. The student would then be tasked with “filling in the blank”, and in doing so is forced to learn the underlying language rules. In the field of computer vision, crops, rotations, and colour distortions are used to generate a transformed view of a sample image, again forcing the student network to learn the important features common to both instances.
Studies have shown that the quality of representations learned can be very sensitive to the choice of transform. Fortunately, a research team at Google was able to leverage its resources to perform a comprehensive study of image-based transforms, providing framework for efficient SSL for computer vision tasks called SimCLR [1].
Crucially, these pairs of sample views can be generated automatically, and with no explicit prior knowledge of the contents of the dataset (beyond an appropriate choice of transform). Further, feature learning is autonomous and task agnostic. Once the self-supervised portion of the training is complete, the teacher network and projection heads can be discarded, and the student network can be applied to the desired task via transfer learning. Here, a new classification layer (or layers) is appended to the network and trained on the task using a labelled dataset. However, having already learned suitable representational encodings during the SSL process, the final (supervised) learning step can be performed with far less labelled data.
SSL therefore presents a new training paradigm that can drastically reduce the cost and manpower that traditionally accompanies the training of complex models on large datasets. For Plextek, this means that more time can be spent on meaningful development, adding functionality and improving the quality of model output. SSL may also make domain-specialised problem spaces more accessible to machine learning approaches, where ordered datasets do not exist, or are under-represented amongst the open-source repositories available online. Underwater acoustics and an increasingly cluttered RF spectrum are two such challenging environments in which Plextek has a history of expertise, and where SSL techniques can not only increase the effective volume of usable training data, but also improve the quality of features learned. This in turn enables the development of powerful, custom ML applications, tailored to the needs of the customer.
Already, models trained under an SSL framework have been demonstrated to perform at an equivalent or better level than their classically trained counterparts. Recently, for example, an SSL method called DINO has been demonstrated by a research team at Meta AI [2], training a vision transformer (ViT) on image recognition tasks. Incredibly, they found that their model displayed an emergent capability for object segmentation, having learned to do so with no prior object labelling or masking.
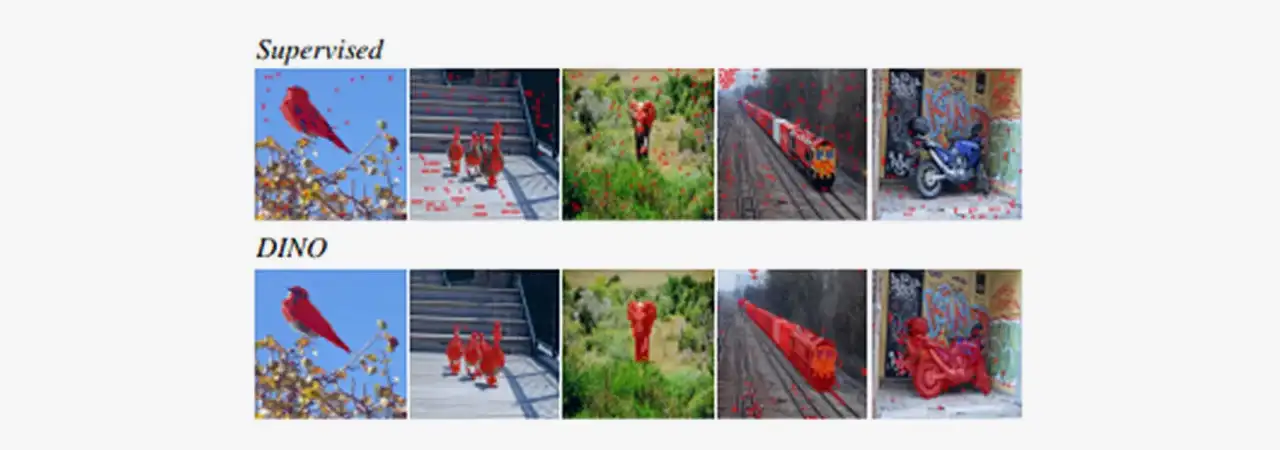
Segmentations learned by equivalent ViT’s under supervised and self-supervised (DINO) learning frameworks
This surprising level of capability, arising naturally from autonomous learning, is one of the reasons SSL has been hailed the “dark matter of intelligence” by Meta’s VP and Chief AI Scientist Yann LeCun. A model’s ability to learn unassisted, by observation of the world around it, is a landmark step on the way to artificial general intelligence (AGI), allowing the model to develop an intuitive understanding of relationships and features within a dataset, before even being set to the desired end-task. This in turn better ensures that its decision-making output is grounded in analysis of the relevant features, making the model more robust to new contexts and environments, quicker to re-train on previously unseen classes, and, perhaps of more interest to those analysts, gives them a day off from labelling yet another endless dataset.
[1] A Simple Framework for Contrastive Learning of Visual Representations, Chen et al, 2020
[2] Emerging Properties in Self-Supervised Vision Transformers, Caron et al, 2021
Technology Platforms
Plextek's 'white-label' technology platforms allow you to accelerate product development, streamline efficiencies, and access our extensive R&D expertise to suit your project needs.
-
01 Configurable mmWave Radar Module
Plextek’s PLX-T60 platform enables rapid development and deployment of custom mmWave radar solutions at scale and pace
-
02 Configurable IoT Framework
Plextek’s IoT framework enables rapid development and deployment of custom IoT solutions, particularly those requiring extended operation on battery power
-
03 Ubiquitous Radar
Plextek's Ubiquitous Radar will detect returns from many directions simultaneously and accurately, differentiating between drones and birds, and even determining the size and type of drone
Downloads
View All Downloads- PLX-T60 Configurable mmWave Radar Module
- PLX-U16 Ubiquitous Radar
- Configurable IOT Framework
- Cost Effective mmWave Radar Devices
- Connected Autonomous Mobility
- Antenna Design Services
- Drone Sensor Solutions for UAV & Counter-UAV Awareness
- mmWave Sense & Avoid Radar for UAVs
- Exceptional technology for marine operations