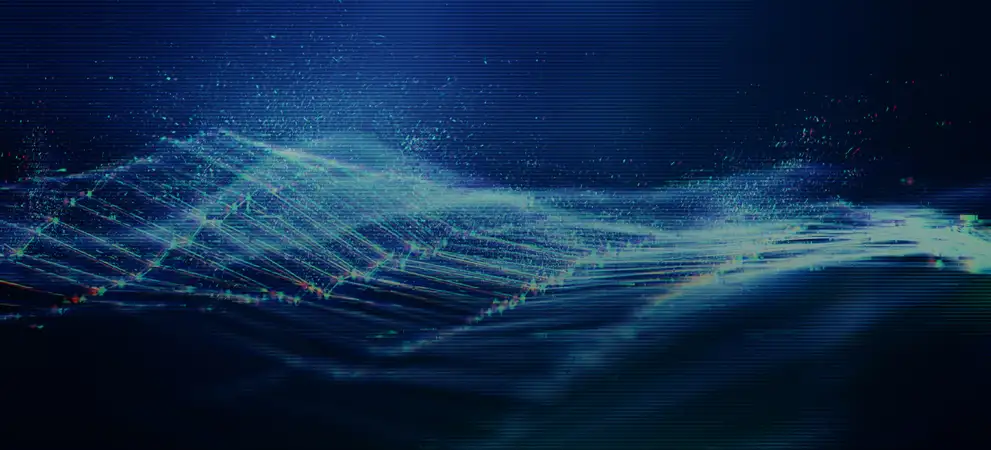
Rapid RF Propagation Modelling
Mapping radio waves in seconds with the help of machine learning
Finding just the right location is crucial for cellular network operators when it comes to maximising the coverage of a new base station.
Radio frequency (RF) propagation modelling comes to the rescue, mapping where transmitter signals will go according to the surroundings – they will bounce off buildings, for example, causing shadow regions and be affected by the terrain.
But traditional physics-based modelling tools can take weeks to derive an optimum location – and that is no use when time is of the essence – e.g. for aid agencies operating in a disaster zone after an earthquake.
That’s where rapid RF propagation modelling comes in – using machine learning (ML) models to crunch the numbers. Once an ML algorithm is trained on data from traditional modelling tools, it can give an answer to a new problem in a fraction of the time and with far less computational resource.
Key skills
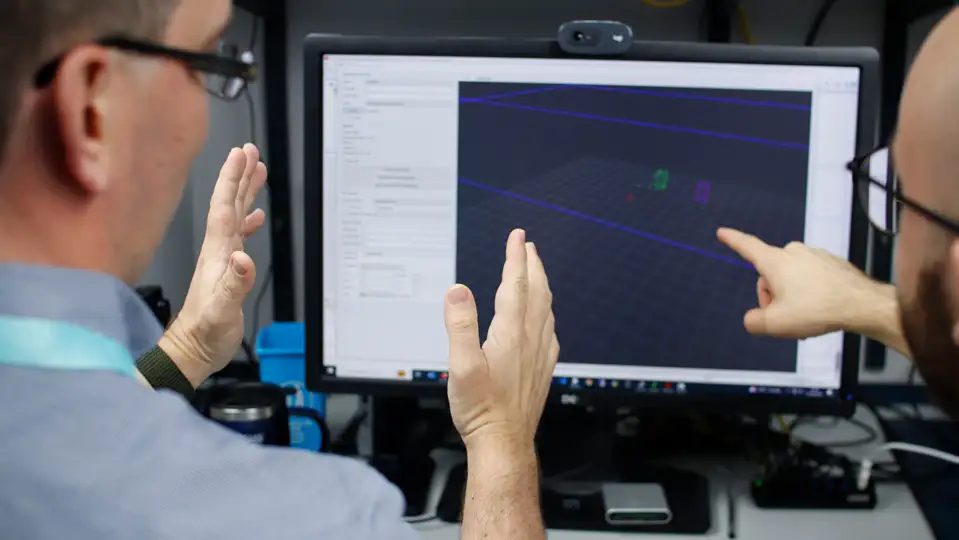
-
ML algorithm development
Creating algorithms capable of learning from data to predict RF propagation patterns efficiently, representing a core technology that powers rapid modelling capabilities.
-
Physics-based RF modelling proficiency
Deep understanding of traditional RF propagation physics to ensure accurate baseline models, crucial for training effective ML models.
-
Data-driven network optimisation
Data analytics to enhance network performance and coverage, ensuring optimal use of resources in any terrain.
-
Cross-terrain signal prediction
Ability to accurately forecast signal behaviour across diverse environments, from urban landscapes to rural settings, enhancing connectivity and network reliability.
-
Computational performance enhancement
Enhancing the speed and efficiency of simulations on standard computing hardware, markedly decreasing the time required for propagation modelling.
-
Emergency communications deployment
Setting up communication networks swiftly in disaster scenarios, a critical component of rapid response efforts.
-
Advanced RF propagation analysis
Conducting assessments of RF propagation to inform the planning and optimisation of communication networks, incorporating complex environmental factors.
What sets us apart when it comes to rapid RF propagation modelling?
Combining Plextek’s deep knowledge of physics-based principles with our expertise in ML, we can deliver accurate and dependable coverage maps, ensuring rapid deployment of comms networks.
- ML
- RF
- ML-driven RF modelling
- ML in RF Planning
- Emergency response networks
- Real-time coverage mapping
- Scalable network planning
- Propagation modelling
- Efficient communication networks